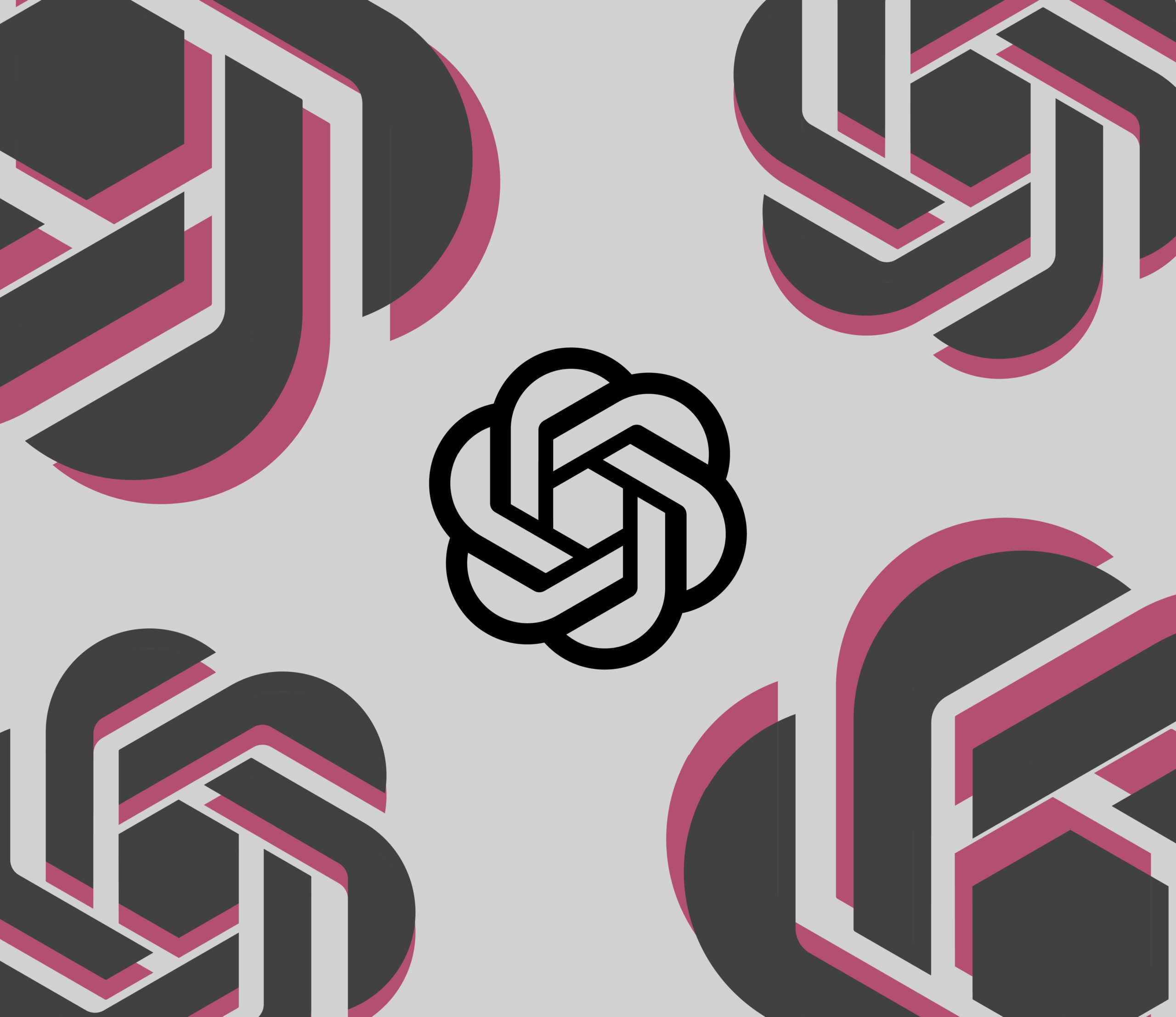
Open AI’s 2023 Developer Conference
And What it Could Mean for the Future of LLMs
Brock Moir
Chief Product Officer, AGvisorPRO Inc.
Note, given the recent turmoil at Open AI, I held off on publishing this article. Now that some stability has been achieved, it seems like full steam ahead.
Following the Open AI developer conference keynotes, let’s take a look at what this means for the future of artificial general intelligence (AGI) and especially the Open AI platform.
Let’s dig in!
Some of the highlights from their release:
GPT4 turbo is now 1/3 the price and 3x the speed! And having 128k tokens of available context is fire! Expect this trend to continue, OpenAI is spending big on infrastructure and seems focussed on driving down their costs while increasing speed and throughput.
Developers will now have more control over completions with formatted json outputs and temperature controls. json is a commonly used data exchange format, having json formatted output allows a developer to force the GPT mode to conform to a specified output structure. json formatted outputs will become critical for most automation tasks developers are using GPTs for. As a platform, Open AI will likely continue adding new ways to control the output of their models so that developers can better leverage GPTs for their use cases.
Custom GPTs are something new, and perhaps to some, expected as it adds a convenient wrapper over Open AI’s models to make it easier to create specialty agents. Developers can now create custom ChatGPT threads with their own personality, custom actions, knowledge retrieval, and access to the code interpreter. The GPT marketplace will enable developers to share and potentially monetize their own GPTs.
A hint at multimodal capabilities, GPT-4 can now ‘see’ and ‘hear’ by giving it images or audio input. It can also generate robust speech patterns and images. However, their image generation still leaves a lot to be desired. In the future leveraging multimodal embeddings from a common model may offer a powerful way to search through different types of data. As we move to more AI enabled human-computer interfaces, multimodal capabilities will help the AI experience feel more natural.
Custom, fine-tuned GPT4 models, for a cool ~$3 million you can fine tune a version of GPT4 in your unique domain.
What could this mean for the future AI platforms?
An evolution in the human-computer interface
AI enabled assistants or GPTs open up a new opportunity for the evolution of the human-computer interface. We have seen hints of this before with Google Home, Siri, Alexa, etc; GPTs can put these devices on steroids. Now, not only can the AI better understand what you are asking; but it can accomplish a much wider variety of tasks while providing you with responses based on unstructured data.
I expect OpenAI to build into a more formal marketplace of custom actions and GPTs that any developer can build and purchase. Similar to the app store, OpenAI will have some control of the quality, provide for some form of transaction, and take a cut of the revenue.
Developers that leverage AI based interfaces will find significant competitive advantages in usability, speed in information retrieval, and ability to leverage otherwise unstructured inputs; all of which will create competitive advantages to the early movers in this space.
Custom tuned GPT models for repeatable superhuman task completion
Assistants basically merge custom prompts and retrieval augmented generation, which is super powerful, but under the hood it is still ‘just’ GPT-4. One of the announcements this year was in the ability to fine tune GPT-4 for domain specific use cases; it is yet to be understood how valuable this may be beyond GPT-4’s capabilities.
However, there are many valuable use cases that can be unlocked by taking parts of the GPT-4 model and retraining it to do specific tasks. Open AI has not mentioned this, but it seems like a likely area of differentiation for them to pursue.
As an example of what I mean here is; in the retrieval augmented generation space, we use the GPT-4 embeddings to match input to the best section to reference using cosine similarity. This method is simple and effective, but it doesn’t do a great job of helping us understand why that section was relevant, or even really which sections may be the most relevant (when given similar sections or more vague input queries). However, a custom model that leverages the first few layers of GPT-4 could easily just output these sorts of things (GPT-4 could, but it becomes cost prohibitive to use in this regard).
I expect OpenAI to build custom models based on some of the most common tasks that developers perform (for example retrieval augmented generation), and fine tune more compact models that can perform these tasks at super-human capabilities and at a cheaper price point than running the query through GPT-4.
All in all, the AI enabled future is looking bright. For developers that learn how to redesign workflows that leverage these tools, there is potential for significant competitive advantages. As I continue to say, this is lightning in a bottle; and those bold enough to move fast will be able to leverage it with significant upside.