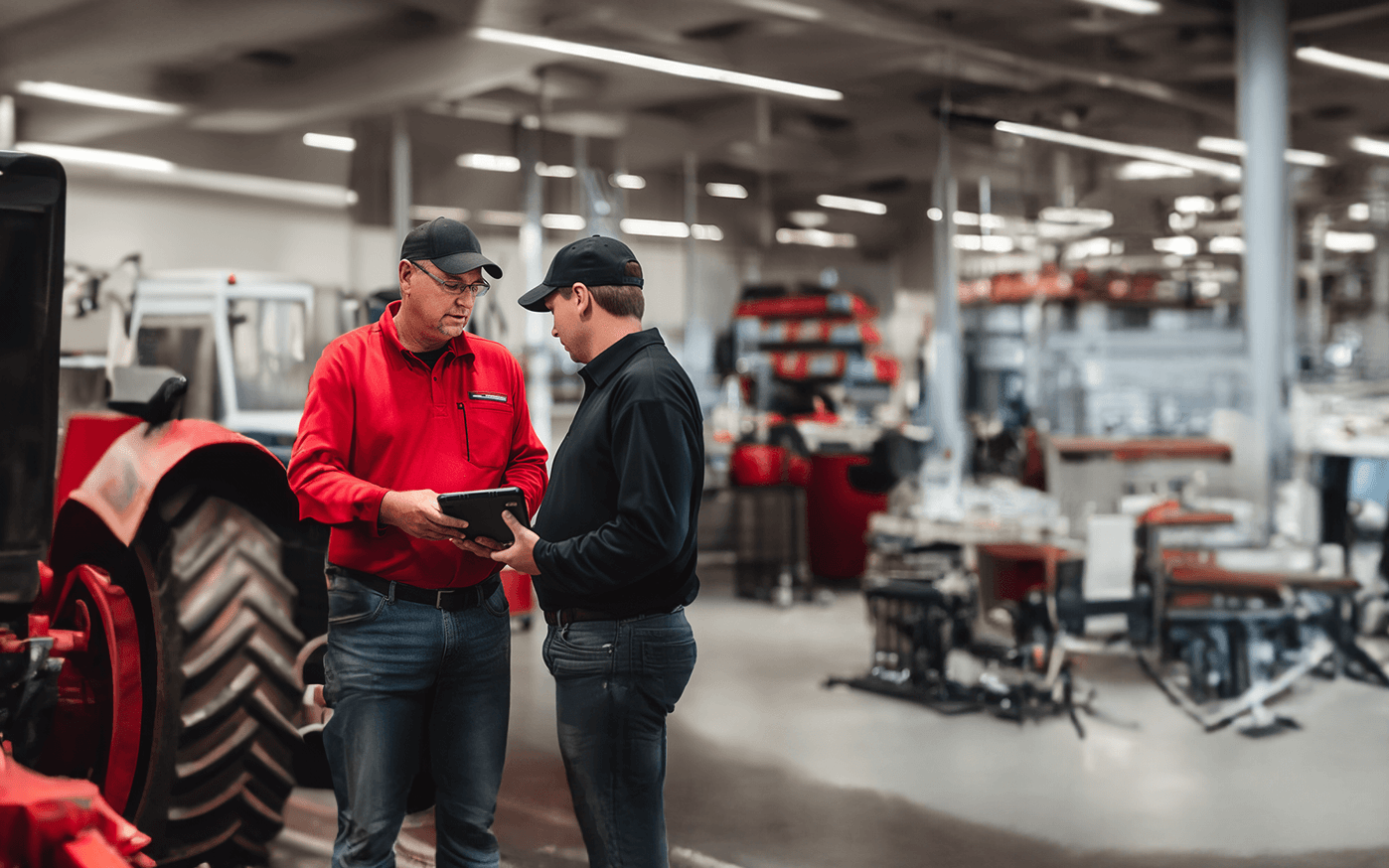
Cultivating Relationships and Nurturing Knowledge
Why Large Language Models Will Transform Agriculture
Brock Moir
Chief Product Officer, AGvisorPRO Inc.
The latest wave of generative AI has advanced to the point that it is able to accomplish tasks traditionally reserved for human experts. Given that I am currently interested in building products enabled by a specific type of generative AI within a certain market, namely Large Language Models (LLMs) in Agriculture, I decided it would be a good idea to collect some thoughts on this intersection. This article will delve into the intricacies of LLMs, their potential benefits and limitations, and how they are set to reshape parts of the agricultural industry.
LLMs are a class of generative AI that can create text-based outputs. The best of these models are trained on all of the text-based data that is openly available on the internet. As a result, the generated output follows the rules and patterns ingrained within our knowledge sources. This gives LLMs the impressive property that they are able to generate impressive outputs that make them appear to have an expert-level understanding of many fields.
This new wave of AI enables solutions to significant and valuable problems that were previously not feasible with prior models. For LLMs, the class of solutions that are newly enabled lie where organizations have language-based interfaces with their customers and employees. Think marketing, sales, service, support, operations, legal, etc. LLMs have the potential to transform most of these functions in very significant ways.
How do LLMs work?
In the simplest sense, almost all versions of machine learning can be summed up as prediction machines; they are machines created solely to produce a new prediction when given a new example.
Generative models are not an exception to this. In the case of LLMs, given some input text it will try to predict the most likely next series of words. With today’s models, its output is similar to human expert-level completions to the point where it seems like the ‘next series of words’ were written by a human rather than a machine.
The exceptional nature seen in the latest generation of LLMs mainly comes from the amount of data used to train the model and the size of the models. Not only are OpenAI’s GPT models very large compared to other AI systems (containing trillions of parameters), but they are also assumed to be trained on most of the openly available text on the internet. The cost of training these models is enormous, purportedly costing OpenAI hundreds of millions of dollars to build and train.
Its seemingly intelligent responses are nothing more than the model making a prediction about what should follow given what has already been said. It does this based on the language relationships it has learned from the knowledge available on the internet.
Who will win with LLMs?
The short answer is that everyone will win with LLMs.
The longer answer is that it is complicated …
LLMs aren’t good at everything. They are designed to always complete a prompt, as such it is very easy to get it to produce made-up information (for example ask ChatGPT to provide a reference to any question you ask it). Furthermore, they have no conception of their own limitations. Because of these factors, it can be difficult to leverage these models off-the-shelf in reliable ways that can be transformational. These technical constraints make it difficult to understand what class of problems LLMs can help solve. In order to overcome this, organizations need to have technical people at the table making decisions about what problems should be solved with LLMs; otherwise, the organization risks deciding on projects that won’t be technically feasible, or trustworthy enough for the end user to utilize confidently.
The reality is, most traditional organizations aren’t very far along in their digital transformation. This limitation will make it difficult for the majority of organizations to identify transformational opportunities for LLMs. Likely some of these companies may be resistant to solutions enabled by LLMs because those solutions can be disruptive to some parts of their current business model.
With these hurdles in mind, I see a few obvious archetypes to determine organizations leading the way with LLMs:
1. OpenAI, Microsoft, Google and other platform companies
This should be obvious, the companies that build the platforms that other companies will use are going to win big. During the gold rush, the surest bet is to sell gold pans, sluice boxes, and shovels!
2. Large traditional companies that are well underway in their digital strategies
There are a handful of traditional companies that are more advanced in their digital strategies. These companies are well-positioned to lead the way in how LLMs will transform their business models.
3. Startups building entirely new workflows with LLMs
LLMs enable brand-new workflows that were impossible with prior technologies. Those companies that are able to identify meaningful problems to solve and are capable of building LLM-enabled solutions will be poised to transform significant parts of the industry.
4. Existing solution providers integrating LLMs into the solutions they currently provide
Think about traditional CRM systems, marketing platforms, support tools, and data management providers. There are a wide variety of technology solution providers that have an existing market and will be able to deliver less transformative efficiencies and other ancillary benefits from building LLM-based tools into the workflows they already support. Some may design new workflows to solve problems in more impactful ways. However, given the lack of need for innovation and high switching costs, most enterprise solution providers in Ag are unlikely to justify disrupting their own business models.
LLMs in Agriculture
There are two fundamental dynamics in Ag where there is transformational potential for LLMs: how those in Ag build and maintain relationships, and how those in Ag share and disseminate knowledge.
Scaling Relationships
Agriculture is an industry built on relationships. Farmers, retailers, dealerships, service providers, and manufacturers have traditionally built strong relationships through face-to-face discussions and a firm handshake. However, as the industry grows and evolves, maintaining these relationships can become increasingly challenging. Here’s where LLMs can offer a helping hand:
1. Intelligent Customer Interactions: By leveraging LLMs in customer-facing roles, businesses can significantly scale their capacity to interact with their customers. AI can handle routine interactions in an authentic way, which allows technical staff to focus on more complex, personal interactions that strengthen relationships.
2. Augmenting Support and Technicians: The use of LLMs in customer support is another potent strategy. LLMs can help frontline workers find and interpret technical documents and manuals. The result is more efficient support, improved customer satisfaction, and stronger relationships.
3. Enhanced Manufacturer-Retailer Dynamics: For manufacturers, LLMs present an opportunity to scale their relationship management with both distributors and end users. If done well, this will improve service from the manufacturers to both end users and distributors and provide better user insights informing future product development and strategies. Distributors, on the other hand, benefit from the enhanced service and support from manufacturers, and by having a superior product to sell and support.
Sharing Knowledge
The agricultural industry contains a significant amount of growing complexity. Whether it’s inputs, equipment, marketing, or logistics; it is growing increasingly challenging to navigate these domains. One major hurdle to scaling this is how knowledge is shared within the industry. Here are a few ways LLMs will contribute in this area:
1. Data Processing and Insights Extraction: There’s an enormous amount of unstructured text data generated in agriculture that previously was not possible to analyze. LLMs can significantly help in mining this data for valuable insights or repurposing it to support new products. For most businesses this could help to inform strategies, optimize operations, and make smarter decisions; for some businesses, this will support entirely new revenue opportunities.
2. Training and Augmentation Tools: LLMs can also be built into support tools for staff, particularly those in technical roles. Most of the technical disciplines in Ag are challenged with retaining institutional knowledge, training new specialists, and finding efficiencies for their expensive technical and support staff. These models can help technical specialists collect, edit, and share knowledge from their organization, making knowledge transfer faster and more efficient.
3. Technical Document Processing: Service providers in Ag can use LLMs to process all kinds of technical documents; essentially creating a unique search and summarization experience. This can help disseminate the knowledge encapsulated in the documents and improve the overall service provided to farmers.
While the age-old principles of farming won’t change, the way we build relationships and share knowledge in agriculture is poised for a significant transformation. However, to unlock the real value of LLMs, businesses need to control their own digital destiny, which means thinking strategically and investing wisely in digital transformation initiatives. Failing to do so could mean missing out on the incredible opportunities LLMs present.
Conclusion
Large Language Models mark a new era of technological disruption and potential growth. Their capacity to generate text-based outputs has substantial implications for various industries.
Not everyone will succeed in building LLM-enabled solutions, however, almost everyone will find LLMs transformative to the way they do business. To successfully harness the potential of LLMs, organizations must be ready to evolve their digital strategies and be open to redefining their business models. As the LLM era unfolds, it will be the innovators and early adopters, those willing to embrace and adapt to this technological shift, who will emerge as winners in their respective fields.